By Amrik Sen | Financial Express | September 21, 2021
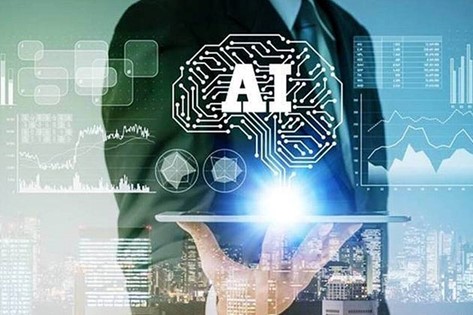
Epidemiological forecasts and smart testing strategies are not the only avenues where AI can play a major role in future pandemics.
A detailed mathematical analysis of probability matrices using AI help countries to get predictive capabilities about the changing landscape of the epidemic over time.
Artificial Intelligence (AI) companies like BlueDot and Metabiota were able to correctly identify unusual cases of pneumonia in Wuhan, China more than a week before the World Health Organization (WHO) formally warned about the impending COVID-19 epidemic.
Interestingly, these American companies were situated far away from the epicentre. They relied on local Chinese news outlets and health reports as data for their sophisticated Natural Language Processing (NLP) algorithms to make these early predictions.
However, predicting the evolutionary course of a pandemic becomes increasingly difficult, as the pandemic progresses in time and geography, due to lack of availability of reliable data. Effective surveillance at the epicentre of a potential outbreak becomes imperative. The challenge is that often the location of an outbreak is a moving target.
Future technologies will include detecting pathogenic agents at the most vulnerable of places, eg. hospitals and clinics, in a round the clock manner. Especially for airborne diseases, bio-degradable sticker based patches will be affixed on the outer surfaces of masks, face shields and PPE suits of frontline health workers that will collect airborne pathogens periodically.
The intensity of pathogenic material captured by these devices will serve as an indicator for the severity of the epidemic prevailing at that time in the immediate geographical neighbourhood. This data will be stored dynamically in a centralised cloud-based server and will populate the probability entries of a certain stochastic matrix. The severity level of the prevailing disease outbreak (eg. mild, moderate or severe) will constitute the state space of this stochastic matrix. The entries of this matrix will denote the transition probabilities between any two states (severity levels).
In this manner, a provincial model of the epidemic can be developed in the form of these probability matrices using a computer. A detailed mathematical analysis of such matrices using AI will give us predictive capabilities about the changing landscape of the epidemic over time. Provincial heat maps can be constructed based on the predictions of this stochastic model and resource optimisation (eg. drug and vaccine delivery, availability of PPE kits for health workers, oxygen supply, etc.) can be conducted by public health administrators in a scientifically cohesive and efficient manner. This will reduce resource wastage.
Further, in many countries, and especially while under duress from a surge in caseloads, effective testing strategies become paramount. One of the successful methodologies is to perform pool testing whence many samples are mixed together and tested. If such a pooled test comes negative, then all patient samples in the specific pool may be deemed to have tested negative.
Concomitantly, machine learning algorithms can be fed with demographic, personal and historical (eg. travel history of people) data to select optimal grouping strategies for pool testing by winnowing out high risk individuals. Pool testing with low risk individuals will speed up testing and increase its efficiency.
Thus, AI based machine learning algorithms can be effectively used in tandem with pool-testing methods to scale up testing and quickly expand the scope of disease surveillance.
Epidemiological forecasts and smart testing strategies, described above, are not the only avenues where AI can play a major role in future pandemics. Computational models of protein structures are often based on state of the art AI technologies that will play a leading role in drug design and delivery.
For example, a free modelling methodology based on deep learning techniques of the AlphaFold system of DeepMind (a Google subsidiary) was successfully used in predicting protein folding mechanisms, without relying on prior templates, to design new drugs that neutralised the viral proteins. Such technologies enable rapid discovery and prototyping of drugs 4 and vaccines that are necessary in the event of a sudden epidemic outbreak.
One of the frustrating unknowns about a pandemic like the COVID-19 is the unpredictable course of disease progression in affected people — while some patients have managed to escape with mild symptoms, others with seemingly similar physical traits have suffered from severe disease.
At the peak of an outbreak, this unknown factor makes the task of doctors difficult in prioritising treatment to patients presenting themselves at hospitals with SARS-CoV2 symptoms, and especially those who will progress to severe disease eventually and may have benefitted from early aggressive intervention. AI based technologies will play a major role in early prognosis of severe disease in patients that will in turn enable medical practitioners to undertake scientifically informed decisions about the treatment strategy for individual patients. This will save many 5 precious lives.
As history suggests, intermittent occurrences of epidemics are something we will have to deal with in the foreseeable future. In such scenarios, what will progressively become a game changer in our ability to effectively combat the disease is an effective multi-pronged approach.
This will include artificial intelligence technologies powered by state of the art mathematical models, reliable data, and effective surveillance mechanisms.
(The author is Faculty, Plaksha University. Views expressed are personal and do not reflect the official position or policy of the Financial Express Online).
This article was first published in Financial Express on September 21, 2021.